Voting Classifier
Suppose we have trained a few classifiers, each one achieving about 80% accuracy.
A very simple way to create an even better classifier is to aggregate the predictions of each classifier and predict the class that gets the most votes.
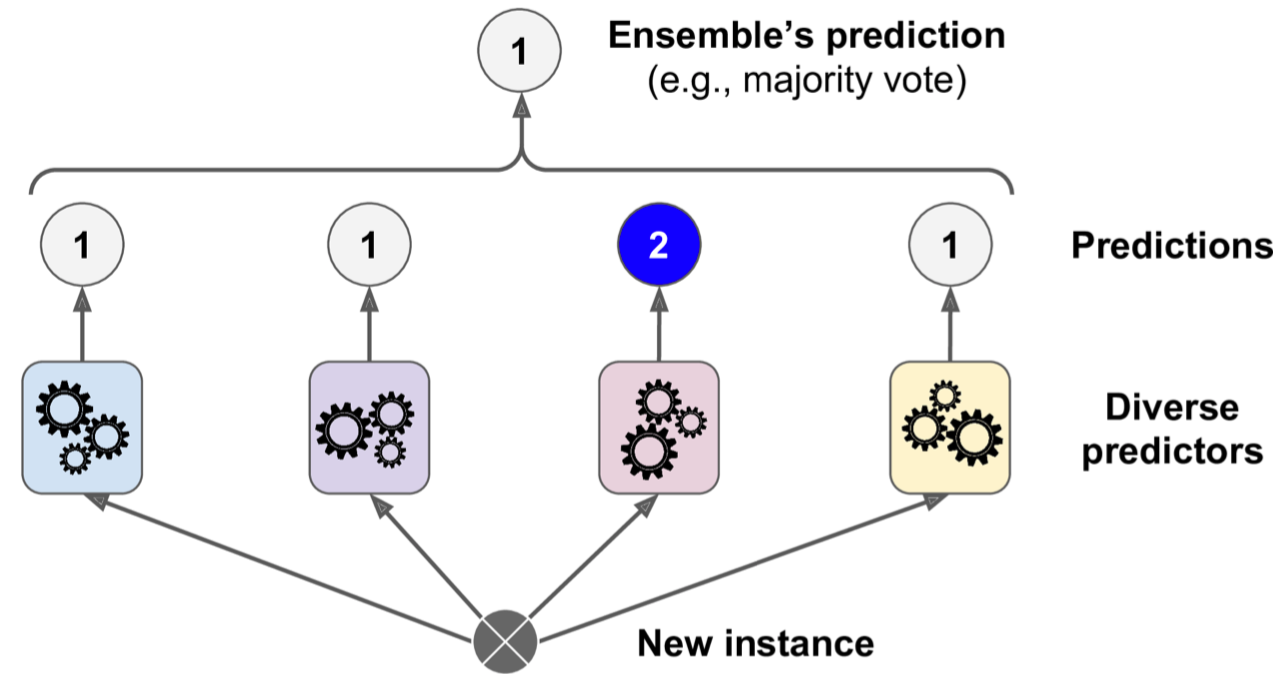
This majority-vote classifier is called a hard voting classifier
Surprisingly, this voting classifier often achieves a higher accuracy than the best classifier in the ensemble. In fact, even if each classifier is a weak learner (meaning it does only slightly better than random guessing), the ensemble can still be a strong learner (achieving high accuracy), provided there are a sufficient number of weak learners and they are sufficiently diverse. (Reason behind: the law of large numbers)
Ensemble methods work best when the predictors are as independent from one another as possible.
- One way to get diverse classifiers is to train them using very different algorithms. This increases the chance that they will make very different types of errors, improving the ensemble’s accuracy.
- Another approach is to use the same training algorithm for every predictor, but to train them on different random subsets of the training set. (See Bagging and Pasting)